Privacy-Preserving Machine Learning. A use-case-driven approach to building and protecting ML pipelines from privacy and security threats Srinivasa Rao Aravilli, Sam Hamilton

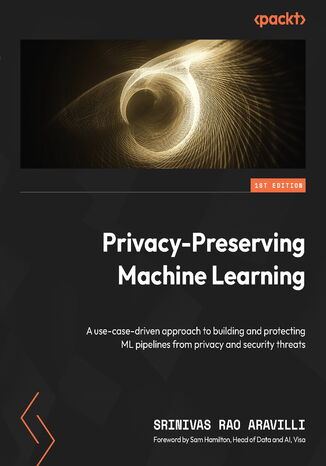
- Autorzy:
- Srinivasa Rao Aravilli, Sam Hamilton
- Serie wydawnicze:
- Learning
- Wydawnictwo:
- Packt Publishing
- Ocena:
- Stron:
- 402
- Dostępne formaty:
-
PDFePub
Opis
książki
:
Privacy-Preserving Machine Learning. A use-case-driven approach to building and protecting ML pipelines from privacy and security threats
– Machine learning engineers face the dual challenge of analyzing vast amounts of data for insights while protecting sensitive information
– This book addresses the complexities arising from large data volumes and the scarcity of in-depth privacy-preserving machine learning expertise, and covers a comprehensive range of topics from data privacy and machine learning privacy threats to real-world privacy-preserving cases
– As you progress, you’ll be guided through developing anti-money laundering solutions using federated learning and differential privacy
– Dedicated sections will explore data in-memory attacks and strategies for safeguarding data and ML models
– You’ll also explore the imperative nature of confidential computation and privacy-preserving machine learning benchmarks, as well as frontier research in the field
– Upon completion, you’ll possess a thorough understanding of privacy-preserving machine learning, equipping them to effectively shield data from real-world threats and attacks
Wybrane bestsellery
Zobacz pozostałe książki z serii Learning
Packt Publishing - inne książki
Dzięki opcji "Druk na żądanie" do sprzedaży wracają tytuły Grupy Helion, które cieszyły sie dużym zainteresowaniem, a których nakład został wyprzedany.
Dla naszych Czytelników wydrukowaliśmy dodatkową pulę egzemplarzy w technice druku cyfrowego.
Co powinieneś wiedzieć o usłudze "Druk na żądanie":
- usługa obejmuje tylko widoczną poniżej listę tytułów, którą na bieżąco aktualizujemy;
- cena książki może być wyższa od początkowej ceny detalicznej, co jest spowodowane kosztami druku cyfrowego (wyższymi niż koszty tradycyjnego druku offsetowego). Obowiązująca cena jest zawsze podawana na stronie WWW książki;
- zawartość książki wraz z dodatkami (płyta CD, DVD) odpowiada jej pierwotnemu wydaniu i jest w pełni komplementarna;
- usługa nie obejmuje książek w kolorze.
Masz pytanie o konkretny tytuł? Napisz do nas: sklep@helion.pl
Książka drukowana

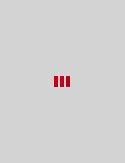
Oceny i opinie klientów: Privacy-Preserving Machine Learning. A use-case-driven approach to building and protecting ML pipelines from privacy and security threats Srinivasa Rao Aravilli, Sam Hamilton
(0)