Practicing Trustworthy Machine Learning Yada Pruksachatkun, Matthew Mcateer, Subho Majumdar

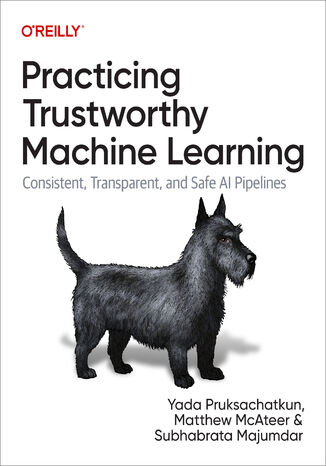
- Autorzy:
- Yada Pruksachatkun, Matthew Mcateer, Subho Majumdar
- Wydawnictwo:
- O'Reilly Media
- Ocena:
- Stron:
- 302
- Dostępne formaty:
-
ePubMobi
Opis
książki
:
Practicing Trustworthy Machine Learning
With the increasing use of AI in high-stakes domains such as medicine, law, and defense, organizations spend a lot of time and money to make ML models trustworthy. Many books on the subject offer deep dives into theories and concepts. This guide provides a practical starting point to help development teams produce models that are secure, more robust, less biased, and more explainable.
Authors Yada Pruksachatkun, Matthew McAteer, and Subhabrata Majumdar translate best practices in the academic literature for curating datasets and building models into a blueprint for building industry-grade trusted ML systems. With this book, engineers and data scientists will gain a much-needed foundation for releasing trustworthy ML applications into a noisy, messy, and often hostile world.
You'll learn:
- Methods to explain ML models and their outputs to stakeholders
- How to recognize and fix fairness concerns and privacy leaks in an ML pipeline
- How to develop ML systems that are robust and secure against malicious attacks
- Important systemic considerations, like how to manage trust debt and which ML obstacles require human intervention
Wybrane bestsellery
O'Reilly Media - inne książki
Dzięki opcji "Druk na żądanie" do sprzedaży wracają tytuły Grupy Helion, które cieszyły sie dużym zainteresowaniem, a których nakład został wyprzedany.
Dla naszych Czytelników wydrukowaliśmy dodatkową pulę egzemplarzy w technice druku cyfrowego.
Co powinieneś wiedzieć o usłudze "Druk na żądanie":
- usługa obejmuje tylko widoczną poniżej listę tytułów, którą na bieżąco aktualizujemy;
- cena książki może być wyższa od początkowej ceny detalicznej, co jest spowodowane kosztami druku cyfrowego (wyższymi niż koszty tradycyjnego druku offsetowego). Obowiązująca cena jest zawsze podawana na stronie WWW książki;
- zawartość książki wraz z dodatkami (płyta CD, DVD) odpowiada jej pierwotnemu wydaniu i jest w pełni komplementarna;
- usługa nie obejmuje książek w kolorze.
Masz pytanie o konkretny tytuł? Napisz do nas: sklep@helion.pl
Książka drukowana

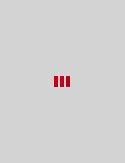
Oceny i opinie klientów: Practicing Trustworthy Machine Learning Yada Pruksachatkun, Matthew Mcateer, Subho Majumdar
(0)