Privacy-Preserving Machine Learning. A use-case-driven approach to building and protecting ML pipelines from privacy and security threats

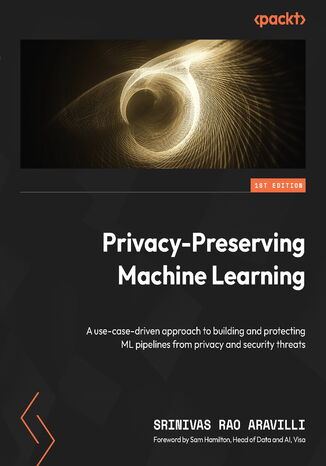
- Wydawnictwo:
- Packt Publishing
- Ocena:
- Stron:
- 402
- Dostępne formaty:
-
PDFePub
Opis
książki
:
Privacy-Preserving Machine Learning. A use-case-driven approach to building and protecting ML pipelines from privacy and security threats
This book delves into data privacy, machine learning privacy threats, and real-world cases of privacy-preserving machine learning, as well as open-source frameworks for implementation. You’ll be guided through developing anti-money laundering solutions via federated learning and differential privacy. Dedicated sections also address data in-memory attacks and strategies for safeguarding data and ML models. The book concludes by discussing the necessity of confidential computation, privacy-preserving machine learning benchmarks, and cutting-edge research.
By the end of this machine learning book, you’ll be well-versed in privacy-preserving machine learning and know how to effectively protect data from threats and attacks in the real world.
Wybrane bestsellery
Packt Publishing - inne książki
Dzięki opcji "Druk na żądanie" do sprzedaży wracają tytuły Grupy Helion, które cieszyły sie dużym zainteresowaniem, a których nakład został wyprzedany.
Dla naszych Czytelników wydrukowaliśmy dodatkową pulę egzemplarzy w technice druku cyfrowego.
Co powinieneś wiedzieć o usłudze "Druk na żądanie":
- usługa obejmuje tylko widoczną poniżej listę tytułów, którą na bieżąco aktualizujemy;
- cena książki może być wyższa od początkowej ceny detalicznej, co jest spowodowane kosztami druku cyfrowego (wyższymi niż koszty tradycyjnego druku offsetowego). Obowiązująca cena jest zawsze podawana na stronie WWW książki;
- zawartość książki wraz z dodatkami (płyta CD, DVD) odpowiada jej pierwotnemu wydaniu i jest w pełni komplementarna;
- usługa nie obejmuje książek w kolorze.
Masz pytanie o konkretny tytuł? Napisz do nas:
Książka drukowana

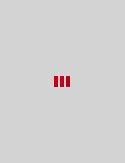
Oceny i opinie klientów: Privacy-Preserving Machine Learning. A use-case-driven approach to building and protecting ML pipelines from privacy and security threats Srinivas Rao Aravilli, Sam Hamilton (0)
Weryfikacja opinii następuję na podstawie historii zamówień na koncie Użytkownika umieszczającego opinię. Użytkownik mógł otrzymać punkty za opublikowanie opinii uprawniające do uzyskania rabatu w ramach Programu Punktowego.