Causal Inference and Discovery in Python. Unlock the secrets of modern causal machine learning with DoWhy, EconML, PyTorch and more

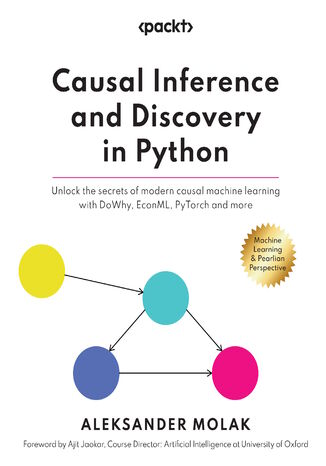
- Wydawnictwo:
- Packt Publishing
- Ocena:
- Stron:
- 456
- Dostępne formaty:
-
PDFePub
Opis
książki
:
Causal Inference and Discovery in Python. Unlock the secrets of modern causal machine learning with DoWhy, EconML, PyTorch and more
You’ll start with basic motivations behind causal thinking and a comprehensive introduction to Pearlian causal concepts, such as structural causal models, interventions, counterfactuals, and more. Each concept is accompanied by a theoretical explanation and a set of practical exercises with Python code. Next, you’ll dive into the world of causal effect estimation, consistently progressing towards modern machine learning methods. Step-by-step, you’ll discover Python causal ecosystem and harness the power of cutting-edge algorithms. You’ll further explore the mechanics of how “causes leave traces” and compare the main families of causal discovery algorithms. The final chapter gives you a broad outlook into the future of causal AI where we examine challenges and opportunities and provide you with a comprehensive list of resources to learn more.
By the end of this book, you will be able to build your own models for causal inference and discovery using statistical and machine learning techniques as well as perform basic project assessment.
Wybrane bestsellery
Packt Publishing - inne książki
Dzięki opcji "Druk na żądanie" do sprzedaży wracają tytuły Grupy Helion, które cieszyły sie dużym zainteresowaniem, a których nakład został wyprzedany.
Dla naszych Czytelników wydrukowaliśmy dodatkową pulę egzemplarzy w technice druku cyfrowego.
Co powinieneś wiedzieć o usłudze "Druk na żądanie":
- usługa obejmuje tylko widoczną poniżej listę tytułów, którą na bieżąco aktualizujemy;
- cena książki może być wyższa od początkowej ceny detalicznej, co jest spowodowane kosztami druku cyfrowego (wyższymi niż koszty tradycyjnego druku offsetowego). Obowiązująca cena jest zawsze podawana na stronie WWW książki;
- zawartość książki wraz z dodatkami (płyta CD, DVD) odpowiada jej pierwotnemu wydaniu i jest w pełni komplementarna;
- usługa nie obejmuje książek w kolorze.
Masz pytanie o konkretny tytuł? Napisz do nas: sklep@helion.pl
Książka drukowana

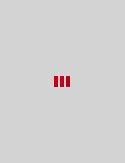
Oceny i opinie klientów: Causal Inference and Discovery in Python. Unlock the secrets of modern causal machine learning with DoWhy, EconML, PyTorch and more Aleksander Molak, Ajit Jaokar (0)
Weryfikacja opinii następuję na podstawie historii zamówień na koncie Użytkownika umieszczającego opinię. Użytkownik mógł otrzymać punkty za opublikowanie opinii uprawniające do uzyskania rabatu w ramach Programu Punktowego.