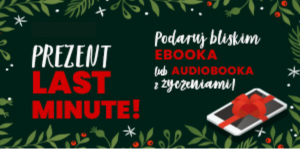
Reliable Machine Learning Cathy Chen, Niall Richard Murphy, Kranti Parisa
(ebook)
(audiobook)
(audiobook)

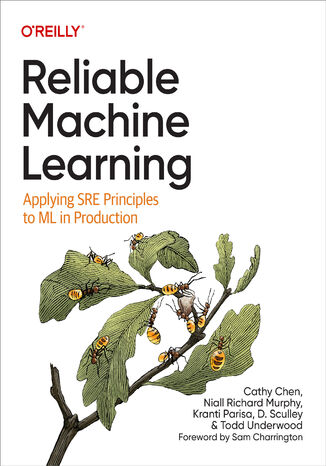
- Autorzy:
- Cathy Chen, Niall Richard Murphy, Kranti Parisa
- Wydawnictwo:
- O'Reilly Media
- Ocena:
- Stron:
- 410
- Dostępne formaty:
-
ePubMobi
Opis
książki
:
Reliable Machine Learning
Whether you're part of a small startup or a multinational corporation, this practical book shows data scientists, software and site reliability engineers, product managers, and business owners how to run and establish ML reliably, effectively, and accountably within your organization. You'll gain insight into everything from how to do model monitoring in production to how to run a well-tuned model development team in a product organization.
By applying an SRE mindset to machine learning, authors and engineering professionals Cathy Chen, Kranti Parisa, Niall Richard Murphy, D. Sculley, Todd Underwood, and featured guest authors show you how to run an efficient and reliable ML system. Whether you want to increase revenue, optimize decision making, solve problems, or understand and influence customer behavior, you'll learn how to perform day-to-day ML tasks while keeping the bigger picture in mind.
You'll examine:
- What ML is: how it functions and what it relies on
- Conceptual frameworks for understanding how ML "loops" work
- How effective productionization can make your ML systems easily monitorable, deployable, and operable
- Why ML systems make production troubleshooting more difficult, and how to compensate accordingly
- How ML, product, and production teams can communicate effectively
Wybrane bestsellery
Cathy Chen, Niall Richard Murphy, Kranti Parisa - pozostałe książki
O'Reilly Media - inne książki
Dzięki opcji "Druk na żądanie" do sprzedaży wracają tytuły Grupy Helion, które cieszyły sie dużym zainteresowaniem, a których nakład został wyprzedany.
Dla naszych Czytelników wydrukowaliśmy dodatkową pulę egzemplarzy w technice druku cyfrowego.
Co powinieneś wiedzieć o usłudze "Druk na żądanie":
- usługa obejmuje tylko widoczną poniżej listę tytułów, którą na bieżąco aktualizujemy;
- cena książki może być wyższa od początkowej ceny detalicznej, co jest spowodowane kosztami druku cyfrowego (wyższymi niż koszty tradycyjnego druku offsetowego). Obowiązująca cena jest zawsze podawana na stronie WWW książki;
- zawartość książki wraz z dodatkami (płyta CD, DVD) odpowiada jej pierwotnemu wydaniu i jest w pełni komplementarna;
- usługa nie obejmuje książek w kolorze.
Masz pytanie o konkretny tytuł? Napisz do nas: sklep@helion.pl
Proszę wybrać ocenę!
Proszę wpisać opinię!
Książka drukowana

Proszę czekać...
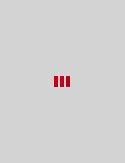
Oceny i opinie klientów: Reliable Machine Learning Cathy Chen, Niall Richard Murphy, Kranti Parisa (0)
Weryfikacja opinii następuję na podstawie historii zamówień na koncie Użytkownika umieszczającego opinię. Użytkownik mógł otrzymać punkty za opublikowanie opinii uprawniające do uzyskania rabatu w ramach Programu Punktowego.