Training Systems using Python Statistical Modeling. Explore popular techniques for modeling your data in Python Curtis Miller

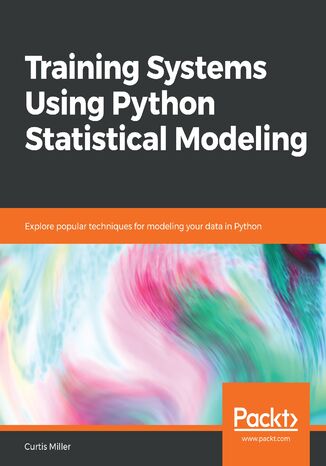
- Autor:
- Curtis Miller
- Wydawnictwo:
- Packt Publishing
- Ocena:
- Stron:
- 290
- Dostępne formaty:
-
PDFePubMobi
Opis
książki
:
Training Systems using Python Statistical Modeling. Explore popular techniques for modeling your data in Python
You’ll start by delving into classical statistical analysis, where you will learn to compute descriptive statistics using pandas. You will focus on supervised learning, which will help you explore the principles of machine learning and train different machine learning models from scratch. Next, you will work with binary prediction models, such as data classification using k-nearest neighbors, decision trees, and random forests. The book will also cover algorithms for regression analysis, such as ridge and lasso regression, and their implementation in Python. In later chapters, you will learn how neural networks can be trained and deployed for more accurate predictions, and understand which Python libraries can be used to implement them.
By the end of this book, you will have the knowledge you need to design, build, and deploy enterprise-grade statistical models for machine learning using Python and its rich ecosystem of libraries for predictive analytics.
Wybrane bestsellery
Packt Publishing - inne książki
Dzięki opcji "Druk na żądanie" do sprzedaży wracają tytuły Grupy Helion, które cieszyły sie dużym zainteresowaniem, a których nakład został wyprzedany.
Dla naszych Czytelników wydrukowaliśmy dodatkową pulę egzemplarzy w technice druku cyfrowego.
Co powinieneś wiedzieć o usłudze "Druk na żądanie":
- usługa obejmuje tylko widoczną poniżej listę tytułów, którą na bieżąco aktualizujemy;
- cena książki może być wyższa od początkowej ceny detalicznej, co jest spowodowane kosztami druku cyfrowego (wyższymi niż koszty tradycyjnego druku offsetowego). Obowiązująca cena jest zawsze podawana na stronie WWW książki;
- zawartość książki wraz z dodatkami (płyta CD, DVD) odpowiada jej pierwotnemu wydaniu i jest w pełni komplementarna;
- usługa nie obejmuje książek w kolorze.
Masz pytanie o konkretny tytuł? Napisz do nas: sklep@helion.pl
Książka drukowana

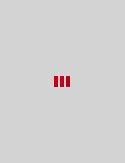
Oceny i opinie klientów: Training Systems using Python Statistical Modeling. Explore popular techniques for modeling your data in Python Curtis Miller (0)
Weryfikacja opinii następuję na podstawie historii zamówień na koncie Użytkownika umieszczającego opinię.